The Nobel Prize in Physics for 2024 was awarded to John Hopfield and Geoffrey Hinton for their transformative work on artificial neural networks, which are the foundation of contemporary machine learning. Their research has enabled breakthroughs in various fields by allowing machines to recognize patterns and learn autonomously, a capability central to the advancements in artificial intelligence today.
The scientists were honored “for foundational discoveries and inventions that enable machine learning with artificial neural networks”, said the Royal Swedish Academy of Sciences.
“The laureates’ work has already been of the greatest benefit. In physics we use artificial neural networks in a vast range of areas, such as developing new materials with specific properties,” said Ellen Moons, chair of the Nobel Committee for Physics.
The Nobel prize, awarded 117 times, comes with a cash award of 11 million Swedish kronor (about $1 million), funded by the bequest of Alfred Nobel, the Swedish inventor who established the award. The laureates will be honored at a ceremony on December 10, marking the anniversary of Nobel’s death.
Last year’s physics Nobel Prize was awarded to three scientists who provided the first-ever split-second glimpse into the ultra-fast world of spinning electrons—a discovery that could pave the way for advancements in electronics or even new approaches to disease diagnosis.
John Hopfield
John Hopfield, a physicist and computational neuroscientist, is renowned for his development of the Hopfield Network in the early 1980s. This model introduced the concept of associative memory networks, which simulate aspects of human memory and are capable of pattern recognition. The Hopfield Network is particularly significant because it mirrors certain processes in biological neural networks, allowing us to understand how memories are stored and retrieved in the brain.
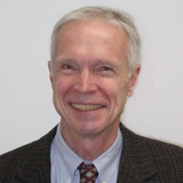
His work laid the groundwork for future advancements in neural networks by demonstrating how complex memory retrieval processes could be modeled mathematically. This contribution has had lasting impacts not just on AI but also on cognitive science and psychology, influencing theories about human memory and cognition.
Geoffrey Hinton
Geoffrey Hinton, often called the “godfather of deep learning,” is a pioneer in developing the foundational concepts that underpin modern neural networks. Hinton’s work with backpropagation algorithms—critical for training deep learning models—has been transformative. These algorithms enabled AI systems to learn from vast datasets, revolutionizing fields like image and speech recognition, natural language processing, and even autonomous driving.
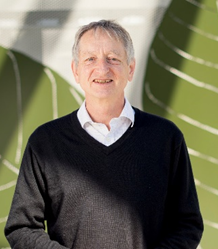
Hinton has consistently pushed the boundaries of what neural networks can achieve. His work has led to breakthroughs such as convolutional neural networks and capsule networks, further expanding the capabilities of machine learning. His influence extends beyond academia; his work has profoundly impacted industry giants like Google, where he has helped steer their AI research, advancing applications that are now part of daily life.
But concerns loom!
AI-powered models can enhance our understanding of complex systems, from climate models to biological processes. By recognizing pioneers in AI, the Nobel Prize opens doors for AI tools to be integrated into diverse research fields, potentially accelerating discovery rates.
However, the recognition of AI in physics through this Nobel Prize brings with it potential threats and challenges. As AI technologies advance, ethical concerns such as biases in algorithms, job losses due to automation, and possible misuse in military or surveillance contexts become increasingly prominent. This award could inadvertently accelerate AI deployment without adequate regulatory oversight, which may exacerbate these issues.
“Collectively, humans carry the responsibility for using this new technology in a safe and ethical way for the greatest benefit of humankind,” the chair of the Nobel Committee for Physics added.
Furthermore, while AI provides powerful tools, there is a risk of over-reliance on its models in scientific research. Integrating AI into physics without fully understanding its limitations could lead to misguided research paths or unwarranted confidence in AI-derived conclusions. Additionally, the convergence of AI and physics may result in intellectual isolation, as traditional physicists might be marginalized in favor of computational methods, potentially diminishing the diversity of perspectives and approaches within scientific inquiry.
Lastly, as Hopfield and Hinton’s groundbreaking work continues to reshape the landscape of AI and physics, the global scientific community faces the dual challenge of harnessing this transformative potential responsibly while navigating the ethical and practical complexities that accompany these advancements.